--
--
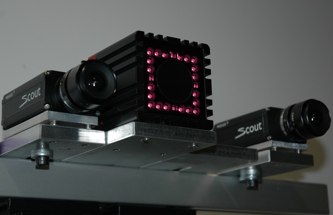
Current 3D video applications require the availability of depth
information, that can be acquired real-time by stereo vision systems
and Time-of-Flight (ToF) cameras. We consider an heterogeneous acquisition system,
made of two high resolution standard cameras L, R (stereo pair) and
one ToF camera T. The stereo system and the ToF camera must be
properly calibrated together in order to jointly operate. We
introduced a generalized multi-camera calibration technique. We
derived a probabilistic fusion algorithm that allow us to obtain
high quality depth information from the information of both the ToF
camera and the stereo-pair.
- Paper [1] and [2] provide a general formalization of the data fusion problem.
The fusion algorithm is derived in a probabilistic setup, that allows
the decoupling of the information from the stereo pair and the
information from the ToF camera. Accurate models for the measurement
errors of the stereo and ToF systems are derived and then used into
the probabilistic fusion framework. Paper [2] presents a simpler
version of the approach based on a local ML optimization. In paper
[1] we introduced a more advanced measurement error model accounting
for the mixed pixels effect together with a global MAP-MRF
optimization scheme using an extended version of Loopy Belief
Propagation with site-dependent labels.
- Paper [3] provides an Amplitude Modulation transmission model for
the ToF camera T.
- Paper [4] provides a different approach for the fusion of the two
data sources that extends the
locally consistent framework used in stereo vision to the case
where two different depth data sources are available.
- Paper [5]
extends the framework of paper [4] by introducing novel confidence
measures for the stereo and ToF data and using them to drive the
locally consistent fusion process
- Paper [6]
further extends the approach by exploiting a Convolutional Neural
Network for the estimation of the confidence information. A novel
synthetic dataset has also been constructed
and used for the training of the deep network.
In order to test the effectiveness of our algorithm, we created datasets with ground-truth, available
here
(dataset of papers 2-3),
here (dataset of paper
4) and here (dataset of paper [6]).
Related Papers:
[1]
C. Dal Mutto, P. Zanuttigh, G.M.Cortelazzo
--------
"Probabilistic
ToF and Stereo Data Fusion Based on Mixed Pixels Measurement Models"
-------- Accepted for
publication on IEEE Transaction on Pattern
Analysis and Machine Intelligence, 2015
-------- [
Paper Page ]
----[2]
C. Dal Mutto, P. Zanuttigh, G.M.Cortelazzo
--------
"A
Probabilistic Approach to ToF and Stereo Data Fusion"
-------- 3DPVT10 (IEEE)
-------- Paris, France,
May 2010.
-------- [
BibRef ] [
Dataset Page ]
----[3]
C. Dal Mutto, P. Zanuttigh, G.M.Cortelazzo
--------
"Accurate 3D Reconstruction by Stereo and ToF Data Fusion"
(BEST PAPER AWARD)
-------- GTTI Meeting
2010
-------- Brescia, Italy,
June 2010.
-------- [
BibRef ] [
Dataset Page ] [
Presentation ]
[4]
C.
Dal Mutto, P. Zanuttigh,
S. Mattoccia,
G.M. Cortelazzo
"Locally Consistent ToF and Stereo
Data Fusion"
ECCV 2012
Workshop on Consumer Depth Cameras for Computer Vision (CDC4CV)
--------Florence, Italy,
October 2012.
-------- [
Dataset Page ]
[5] G. Marin, P. Zanuttigh, S. Mattoccia
"Reliable Fusion of ToF and Stereo Depth Driven by Confidence Measures"
European Conference on Computer Vision (ECCV), 2016
[Paper
Page]
[6]
G. Agresti, L. Minto, G. Marin, P. Zanuttigh
"Deep Learning for Confidence Information in Stereo and ToF Data Fusion"
Accepted for publication at ICCV 2017 workshop on 3D Reconstruction meets Semantics.
[Dataset Page ]
For all the IEEE Publications:
(c) IEEE. Personal use of this
material is permitted. However, permission to reprint/republish
this material for advertising or promotional purposes or for
creating new collective works for resale or redistribution to
servers or lists, or to reuse any copyrighted component of this
work in other works must be obtained from the IEEE.ont>
|